Modelling Transport
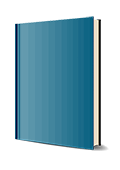
5. Edition March 2024
720 Pages, Hardcover
Textbook
MODELLING TRANSPORT
Comprehensive Textbook Resource for Understanding Transport Modelling
Modelling Transport provides unrivalled depth and breadth of coverage on the topic of transport modelling. Each topic is approached as a modelling exercise with discussion of the roles of theory, data, model specification, estimation, validation, and application. The authors present the state of the art and its practical application in a pedagogic manner, easily understandable to both students and practitioners. An accompanying website hosts a solutions manual.
Sample topics and learning resources included in the work are as follows:
* State-of-the-art developments in the field of transport modelling, including new research and examples
* Factors to consider for better modelling and forecasting
* Information and analysis on dynamic assignment and micro-simulation and model design and specification
* Agent and Activity Based Modelling
* Modelling new modes and services
Graduate students in transportation engineering and planning, transport economics, urban studies, and geography programs along with researchers and practitioners in the transportation and urban planning industry can use Modelling Transport as a comprehensive reference work for a wide array of topics pertaining to this field.
About the Companion Website xxii
1 Introduction 1
1.1 Background 1
1.2 Models and Their Role 2
1.3 Characteristics of Transport Problems 3
1.3.1 Characteristics of Transport Demand 3
1.3.2 Characteristics of Transport Supply 4
1.3.3 A View of Transport Problems 6
1.3.4 A Simple Model 7
1.3.5 Classic and New Modes of Transport 9
1.4 Modelling and Decision-Making 9
1.5 Issues in Transport Modelling 12
1.5.1 General Modelling Issues 12
1.5.1.1 The Roles of Theory and Data 12
1.5.1.2 Model Assumptions 13
1.5.1.3 Model Specification 14
1.5.1.4 Model Calibration, Validation, and Use 15
1.5.1.5 Modelling, Forecasting, and Judgement 16
1.5.2 Aggregate and Disaggregate Modelling 17
1.5.3 Homo Sapiens and Homo Economicus 18
1.5.4 Cross-Section and Time Series 20
1.5.5 Revealed and Stated Preferences 21
1.6 The Structure of the Classic Transport Model 22
1.6.1 The Classic 4/5 Stage Model 22
1.6.2 Granularity 24
1.6.3 Macro, Meso, and Micro Models 27
1.7 Transport Planning and Uncertainty 27
1.8 Theoretical Basis Versus Expedience 31
1.9 Becoming a Better Modeller 33
Exercises 33
2 Data 35
2.1 Basic Sampling Theory 36
2.1.1 Statistical Considerations 36
2.1.1.1 Basic Definitions 36
2.1.1.2 Sample Size to Estimate Population Parameters 38
2.1.1.3 Obtaining the Sample 40
2.1.2 Practical Considerations in Sampling 43
2.1.2.1 The Implementation Problem 43
2.1.2.2 Finding the Size of Each Subpopulation 43
2.2 Errors in Modelling and Forecasting 44
2.2.1 Different Types of Error 45
2.2.1.1 Measurement Errors 45
2.2.1.2 Sampling Errors 46
2.2.1.3 Computational Errors 46
2.2.1.4 Specification Errors 46
2.2.1.5 Transfer Errors 47
2.2.1.6 Aggregation Errors 47
2.2.2 The Model Complexity/Data Accuracy Trade-off 48
2.2.3 Forecasting Errors 51
2.3 Basic Data-collection Methods 53
2.3.1 Practical Considerations 53
2.3.1.1 Length of the Study 53
2.3.1.2 Study Horizon 53
2.3.1.3 Limits of the Study Area 54
2.3.1.4 Study Resources 54
2.3.2 Types of Surveys 54
2.3.2.1 Survey Scope 55
2.3.2.2 Home Interview Travel Surveys 57
2.3.2.3 Other Important Types of Surveys 66
2.3.3 Data Correction, Expansion, and Validation 68
2.3.3.1 Data Correction 69
2.3.3.2 Imputation Methods 71
2.3.3.3 Sample Expansion 72
2.3.3.4 Validation of Results 72
2.3.4 Longitudinal Data Collection 73
2.3.4.1 Basic Definitions 73
2.3.4.2 Representative Sampling 74
2.3.4.3 Sources of Error in Panel Data 75
2.3.4.4 Relative Costs of Longitudinal Surveys 76
2.3.5 Travel Time Surveys 76
2.3.6 Digital Data Sources 77
2.4 Stated Preference Surveys 79
2.4.1 Introduction 79
2.4.1.1 Contingent Valuation and Conjoint Analysis 79
2.4.1.2 Stated Choice Methods 81
2.4.2 The Survey Process 83
2.4.2.1 Clarifying Study Objectives and Defining Objects of Interest 84
2.4.2.2 Defining Experimental Assumptions 86
2.4.2.3 Generating the Experimental Design 92
2.4.2.4 Conduct Post Design Generation Testing 97
2.4.2.5 Conduct Questionnaire 98
2.4.2.6 Nothing is Important 99
2.4.2.7 Realism and Complexity 100
2.4.2.8 Use of Computers in SP Surveys 101
2.4.2.9 Quality Issues in Stated Preference Surveys 102
2.4.3 Case Study Example 103
2.4.4 Limitations of Stated Preference Methods 115
Exercises 115
3 Zones and Networks 119
3.1 Zoning Design 120
3.2 Road Network Representation 122
3.2.1 Traffic Flow 123
3.2.2 Network Details 123
3.3 Link Properties and Functions 125
3.3.1 Link Properties 125
3.3.2 Network Costs 126
3.3.3 Definitions and Notation 127
3.3.4 Speed-Flow and Cost-Flow Curves 127
3.3.5 Public Transport Networks 131
Exercises 132
4 Trip Generation Modelling 133
4.1 Introduction 134
4.1.1 Some Basic Definitions 134
4.1.2 Characterisation of Journeys 135
4.1.2.1 By Purpose 135
4.1.2.2 By Time of Day 135
4.1.2.3 By Person Type 136
4.1.3 Factors Affecting Trip Generation 136
4.1.3.1 Personal Trip Productions 137
4.1.3.2 Personal Trip Attractions 137
4.1.3.3 Freight Trip Productions and Attractions 137
4.1.4 Growth-Factor Modelling 138
4.2 Regression Analysis 139
4.2.1 The Linear Regression Model 139
4.2.2 Zonal-Based Multiple Regression 148
4.2.3 Household-Based Regression 149
4.2.4 The Problem of Non-Linearity 151
4.2.5 Obtaining Zonal Totals 152
4.2.6 Matching Generations and Attractions 153
4.3 Cross-Classification or Category Analysis 153
4.3.1 The Classical Model 153
4.3.1.1 Introduction 153
4.3.1.2 Variable Definition and Model Specification 154
4.3.1.3 Model Application at Aggregate Level 155
4.3.2 Improvements to the Basic Model 156
4.3.2.1 Equivalence Between Category Analysis and Linear Regression 156
4.3.2.2 Regression Analysis for Household Strata 158
4.4 Other Trip Generation Formulations 159
4.4.1 Alternative Model Formulations 159
4.4.1.1 The Negative Binomial (NB) Approach 159
4.4.1.2 The Ordinal Probit Model 160
4.4.1.3 Comparing the Performance of Count Data and Linear Regression Models 160
4.5 Trip Generation and Accessibility 161
4.6 The Frequency Choice Logit Model 162
4.7 Tour Generation 164
4.8 Forecasting Variables in Trip Generation Analysis 165
4.9 Stability and Updating of Trip Generation Parameters 167
4.9.1 Temporal Stability 167
4.9.2 Geographic Stability 168
4.9.3 Bayesian Updating of Trip Generation Parameters 168
Exercises 171
5 Trip Distribution Modelling 173
5.1 Definitions and Notation 174
5.2 Growth-Factor Methods 176
5.2.1 Uniform Growth Factor 176
5.2.2 Singly Constrained Growth-Factor Methods 177
5.2.3 Doubly Constrained Growth Factors 178
5.2.4 Advantages and Limitations of Growth-Factor Methods 180
5.3 Synthetic or Gravity Models 180
5.3.1 The Gravity Distribution Model 180
5.3.2 Singly and Doubly Constrained Models 182
5.4 The Entropy-Maximising Approach 183
5.4.1 Entropy and Model Generation 183
5.4.2 Generation of the Gravity Model 185
5.4.3 Properties of the Gravity Model 187
5.4.4 Production-Attraction Format 189
5.4.5 Segmentation 190
5.5 Calibration of Gravity Models 190
5.5.1 Calibration and Validation 190
5.5.2 Calibration Techniques 191
5.6 The Tri-Proportional Approach 192
5.6.1 Bi-Proportional Fitting 192
5.6.2 A Tri-Proportional Problem 194
5.6.3 Partial Matrix Techniques 195
5.7 Other Synthetic Models 197
5.7.1 Generalisations of the Gravity Model 197
5.7.2 Intervening Opportunities Model 198
5.7.3 Disaggregate Approaches 200
5.8 Practical Considerations 200
5.8.1 Sparse Matrices 200
5.8.2 Treatment of External Zones 201
5.8.3 Special Generators 201
5.8.4 Intra-Zonal Trips 201
5.8.5 Journey Purposes 202
5.8.6 K Factors 202
5.8.7 Adjusting Trip Matrices 203
5.8.8 Errors in Modelling 203
5.8.9 The Stability of Trip Matrices 204
5.8.10 Sense Checks 206
Exercises 206
6 Modal Split and Direct Demand Models 209
6.1 Introduction 209
6.2 Factors Influencing the Choice of Mode 209
6.3 Trip-End Modal-Split Models 211
6.4 Trip Interchange Modal-Split Models 211
6.5 Synthetic Models 213
6.5.1 Distribution and Modal-Split Models 213
6.5.2 Distribution and Modal-Split Structures 215
6.5.3 Multimodal-Split Models 216
6.5.4 Calibration of Binary Logit Models 219
6.5.5 Calibration of Hierarchical Modal-Split Models 220
6.6 Direct Demand Models 222
6.6.1 Introduction 222
6.6.2 Direct Demand Models 222
6.6.3 An Improvement on Direct Demand Modelling 224
6.7 Sense Checks 225
Exercises 227
7 Discrete Choice Models 231
7.1 General Considerations 231
7.2 Theoretical Framework 234
7.3 The Multinomial Logit (MNL) Model 236
7.3.1 Specification Searches 238
7.3.2 Universal Choice Set Specification 239
7.3.3 Some Properties of the MNL 240
7.4 The Nested Logit Model (NL) 241
7.4.1 Correlation and Model Structure 241
7.4.2 Fundamentals of Nested Logit Modelling 242
7.4.2.1 The Model of Williams and of Daly-Zachary 243
7.4.2.2 The Formulation of McFadden: The GEV Family 244
7.4.3 The NL in Practice 246
7.4.3.1 Limitations of the NL 247
7.4.4 Controversies About Some Properties of the NL Model 247
7.4.4.1 Specifications Which Address the Non-Identifiability Problem 247
7.4.4.2 On the Limits of the Structural Parameters 249
7.4.4.3 Two Further Issues 251
7.5 The Multinomial Probit Model 253
7.5.1 The Binary Probit Model 253
7.5.2 Multinomial Probit and Taste Variations 254
7.5.3 Comparing Independent Probit and Logit Models 255
7.6 The Mixed Logit Model 255
7.6.1 Model Formulation 255
7.6.2 Model Specifications 256
7.6.2.1 Basic Formulations 256
7.6.2.2 More Advanced Formulations 258
7.6.3 Identification Problems 259
7.6.3.1 Theoretical Identification 260
7.6.3.2 Empirical Identification 260
7.7 Other Choice Models and Paradigms 261
7.7.1 Other Choice Models 261
7.7.2 Choice by Elimination and Satisfaction 262
7.7.2.1 Compensatory Rule 263
7.7.2.2 Non-Compensatory Rules 263
7.7.3 Habit and Hysteresis 264
7.7.4 Modelling with Panel Data 265
7.7.4.1 Panel Data Models 266
7.7.4.2 Efficiency and Repeated Observations 267
7.7.4.3 Dealing with Temporal Effects 269
7.7.5 Hybrid Choice Models Incorporating Latent Variables 271
7.7.5.1 Modelling with Latent Variables 272
7.7.5.2 Hybrid Discrete Choice Model 272
7.7.6 Attribute Non-Attendance and Other Heuristics 273
Exercises 276
8 Specification and Estimation of Discrete Choice Models 279
8.1 Introduction 279
8.2 Choice-Set Determination 280
8.2.1 Choice-Set Size 280
8.2.2 Choice-Set Formation 281
8.3 Specification and Functional Form 282
8.3.1 Functional Form and Transformations 282
8.3.1.1 Basic Box-Cox Transformation 283
8.3.1.2 Box-Tukey Transformation 283
8.3.2 Theoretical Considerations and Functional Form 283
8.3.3 Intrinsic Non-Linearities: Destination Choice 284
8.4 Statistical Estimation 285
8.4.1 Estimation of Models from Random Samples 285
8.4.1.1 The t-test for Significance of any Component theta¯*k of theta¯* 288
8.4.1.2 The Likelihood Ratio Test 291
8.4.1.3 The Overall Test of Fit 292
8.4.1.4 The rho² Index 293
8.4.1.5 The Percentage Right or First Preference Recovery (FPR) Measure 294
8.4.1.6 Working with Validation Samples 295
8.4.2 Estimation of Models from Choice-based Samples 299
8.4.3 Estimation of Hybrid Choice Models with Latent Variables 300
8.4.4 Comparison of Non-Nested Models 304
8.4.5 Correcting for Endogeneity in Discrete Choice Models 305
8.4.6 Accounting for Stochastic Variables in Choice Models 307
8.4.6.1 Econometric Analysis 309
8.4.6.2 Stochastic Variables Model 310
8.4.6.3 Random Coefficients Model 311
8.5 Estimating the Multinomial Probit Model 311
8.5.1 Numerical Integration 313
8.5.2 Simulated Maximum Likelihood 314
8.5.2.1 The Basic Approach 314
8.5.2.2 Advanced Techniques 315
8.6 Estimating the Mixed Logit Model 317
8.6.1 Classical Estimation 317
8.6.1.1 Estimation of Population Parameters 317
8.6.1.2 Estimating Individual Parameters 318
8.6.2 Bayesian Estimation 319
8.6.3 Choice of a Mixing Distribution 323
8.6.3.1 Alternative Mixing Distributions 324
8.6.3.2 Discrete Mixtures and Latent Class Modelling 325
8.6.3.3 Empirical Identifiability of Latent Class Models 327
8.6.4 Binary Choice Case 327
8.6.5 Random and Quasi Random Numbers 330
8.6.6 Estimation of Panel Data Models 332
8.7 Modelling with Stated-Preference Data 334
8.7.1 Identifying Functional Form 334
8.7.2 Stated Preference Data and Discrete Choice Modelling 336
8.7.2.1 Naive Methods 337
8.7.2.2 Discrete Choice Modelling with Rating Data 339
8.7.2.3 Discrete Choice Modelling with Rank Data 339
8.7.2.4 Modelling with Stated Choice Data 341
8.7.2.5 Model Estimation with Generalised Choice Data 342
8.7.2.6 Modelling with Indifference Alternatives 345
8.7.2.7 Interactions in SC Modelling 351
8.7.2.8 The Problem of Repeated Observations 354
8.7.3 Model Estimation with Mixed SC and RP Data 355
8.7.3.1 Estimation without Considering Correlation among Repeated Observations 355
8.7.3.2 Joint Estimation Considering Correlation between Repeated Observations 358
8.7.3.3 Forecasting with Joint RP-SC Models 359
Exercises 362
9 Model Aggregation and Transferability 365
9.1 Introduction 365
9.2 Aggregation Bias and Forecasting 366
9.3 Confidence Intervals for Predictions 367
9.3.1 Linear Approximation 368
9.3.2 Non-Linear Programming 369
9.4 Aggregation Methods 370
9.5 Model Updating or Transference 373
9.5.1 Introduction 373
9.5.2 Methods to Evaluate Model Transferability 373
9.5.2.1 Test of Model Parameter for Equality 374
9.5.2.2 Disaggregate Transferability Measures 374
9.5.3 Updating with Disaggregate Data 375
9.5.3.1 Updating the Constants 376
9.5.3.2 Updating of Constants and Scale 376
9.5.4 Updating with Aggregate Data 377
Exercises 378
10 Static Assignment 381
10.1 Basic Concepts 381
10.1.1 Introduction 381
10.1.2 Traffic and Queues 383
10.1.3 Factors Influencing Route Choice 385
10.2 Static Traffic Assignment Methods 386
10.2.1 Introduction 386
10.2.2 Modelling Route Choice 387
10.2.3 Tree Building 388
10.3 All-or-Nothing Assignment 390
10.4 Stochastic Methods 392
10.4.1 Simulation-Based Methods 392
10.4.2 Proportional Stochastic Methods 393
10.4.3 Emerging Approaches 395
10.5 Congested Assignment 398
10.5.1 Wardrop's Equilibrium 398
10.5.2 Hard and Soft Speed-Change Methods 400
10.5.3 Incremental Assignment 401
10.5.4 Method of Successive Averages 402
10.5.5 Braess's Paradox 403
10.6 Public-Transport Assignment 405
10.6.1 Introduction 405
10.6.2 Issues in Public-Transport Assignment 405
10.6.2.1 Supply 405
10.6.2.2 Passengers 407
10.6.2.3 Monetary Costs 407
10.6.2.4 The Definition of Generalised Costs 407
10.6.2.5 The Common Lines Problem 408
10.6.2.6 Frequency or Schedule-Based Route Choice 408
10.6.3 Modelling Public-Transport Route Choice 408
10.6.4 Assignment of Public Transport Trips 412
10.6.5 Discrete Route Choice Modelling 413
10.7 Limitations of the Classic Methods 415
10.7.1 The Assumption of Perfect Information about Costs in all Parts of the Network 415
10.7.2 The Assumption that all Movements can be Represented by a Trip Matrix 415
10.7.3 Limitations in the Node-link Model of the Road Network 415
10.7.4 Errors in Defining Average Perceived Costs 416
10.7.5 Not all Trip Makers Perceive Costs in the Same Way 416
10.7.6 Day-to-Day Variations in Demand 417
10.7.7 Imperfect Estimation of Travel Time Changes with Link Flow Changes 417
10.7.8 The Dynamic Nature of Traffic 418
10.7.9 Input Errors 418
10.8 Practical Considerations 418
Exercises 422
11 Dynamic Assignment 425
11.1 Introduction 425
11.2 Travel Time Reliability 425
11.3 Junction Interaction Methods 426
11.4 The Dynamic Nature of Traffic 427
11.4.1 Delays over Time and Space 427
11.4.2 Average and Experienced Travel Times 431
11.5 Dynamic Traffic Assignment (DTA) 432
11.5.1 General Requirements 432
11.5.2 Discretising Time in DTA 433
11.5.3 Micro- and Meso-Simulation 433
11.5.4 Equilibrium and Simulation 435
Exercises 438
12 Equilibrium 439
12.1 Introduction 439
12.2 Equilibrium 439
12.2.1 A Mathematical Programming Approach 440
12.2.2 Social Equilibrium 444
12.2.3 Solution Methods 445
12.2.3.1 The Frank-Wolfe Algorithm 446
12.2.3.2 Route-Based Assignment 447
12.2.3.3 Origin-Based Assignment 448
12.2.4 Stochastic Equilibrium Assignment 450
12.2.5 Congested Public Transport Assignment 451
12.3 Transport System Equilibrium 452
12.3.1 Equilibrium and Feedback 452
12.3.2 Formulation of the Combined Model System 455
12.3.3 Solving General Combined Models 458
12.3.4 Monitoring Convergence 459
Exercises 460
13 Departure Time Choice 463
13.1 Introduction 463
13.2 Macro and Micro Departure Time Choice 463
13.3 Underlying Principles of Micro Departure TIME Choice 464
13.4 Simple Supply/Demand Equilibrium Models 466
13.5 Time of Travel Choice and Equilibrium Assignment 467
13.6 Modelling Disaggregate Time of Day Choice 469
13.7 Joint Mode/Time of Day Choice 474
13.7.1 Data Collection 474
13.7.1.1 Alternatives and Their Attributes 475
13.7.1.2 Stated Preference Data 475
13.7.2 Model Estimation 476
13.7.2.1 Analysis of Results 478
13.7.2.2 Model Valuations 479
13.8 Conclusion 479
14 Complementary Techniques 481
14.1 Introduction 481
14.2 Sketch Planning Methods 482
14.3 Incremental Demand Models 483
14.3.1 Incremental Elasticity Analysis 484
14.3.2 Incremental or Pivot-Point Modelling 485
14.4 Model Estimation From Traffic Counts 488
14.4.1 Introduction 488
14.4.2 Route Choice and Matrix Estimation 489
14.4.3 Transport Model Estimation from Traffic Counts 489
14.4.4 Matrix Adjustments Using Traffic Counts 492
14.4.5 Traffic Counts and Matrix Estimation 497
14.4.5.1 Independence 497
14.4.5.2 Inconsistency 498
14.4.6 Limitations of ME 2 499
14.4.7 Improved Matrix Estimation Models 501
14.4.8 Treatment of Non-Proportional Assignment 502
14.4.9 Quality of Matrix Estimation Results 503
14.4.10 Estimation of Trip Matrix and Mode Choice 504
14.4.10.1 Simple Unimodal Case 504
14.4.10.2 Updating with Aggregate Modal Shares 505
14.4.10.3 Updating with Traffic Counts 505
14.4.10.4 Updating with Combined Information 505
14.5 Gaming Simulation 506
Exercises 508
15 Freight Demand Models 511
15.1 A Subject of Increasing Importance 511
15.2 Factors Affecting Goods Movements 512
15.3 Pricing Freight Services 513
15.4 Data Collection for Freight Studies 514
15.5 Aggregate Freight Modelling 515
15.5.1 Freight Generations and Attractions 516
15.5.2 Distribution Models 516
15.5.3 Mode Choice 518
15.5.4 Assignment 518
15.5.5 Equilibrium 519
15.5.6 Freight and Service Trips 520
15.6 Disaggregate Approaches 521
15.7 Conclusions 522
16 Activity-Based Models 523
16.1 Introduction 523
16.2 Activities, Tours, and Trips 524
16.3 Tours, Individuals, and Representative Individuals 527
16.4 Agent-Based Modelling 528
16.5 Activity-Based Modelling 529
16.5.1 Introduction 529
16.5.2 Population Synthesis 530
16.5.3 Monte Carlo and Probabilistic Processes 533
16.5.4 Structuring, Activities, and Tours 533
16.5.5 Solving ABM 535
16.5.6 Integration with Assignment 536
16.6 Refining Activity or Tour-Based Models 537
16.6.1 Choice of Usual Place of Work and Education 537
16.6.2 Car Ownership 537
16.6.3 In and Out of Home Activities 538
16.6.4 Person Day-Patterns Linked Across Household Members 538
16.6.5 Activities Allocated Explicitly Among Members of the Household 538
16.6.6 Number of Zones Used 538
16.6.7 Time Periods and Time Constraints 538
16.6.8 Network Equilibrium 539
16.7 Challenges of Activity-Based Models 539
16.8 Extending Random Utility Approaches 540
17 Model Design 541
17.1 Introduction 541
17.2 Accuracy and Precision 542
17.3 Model Specification 543
17.3.1 Model Objectives 543
17.3.2 Identify Possible Interventions 544
17.3.3 Identify Relevant Behavioural Responses 544
17.3.4 Technical Specification and Data Requirements 545
17.3.5 Quality Assurance 546
17.4 Model Calibration and Validation 547
17.5 Model Review 548
17.6 Plan Making 548
17.7 Dealing with Uncertainty 550
18 Key Parameters, Planning Variables, and Value Functions 553
18.1 Forecasting Planning Variables 553
18.1.1 Introduction 553
18.1.2 Use of Official Forecasts 554
18.1.3 Forecasting Population and Employment 555
18.1.3.1 Trend Extrapolation 555
18.1.3.2 Cohort Survival 555
18.1.3.3 Transitional Probabilities 556
18.1.3.4 Economic Base 556
18.1.3.5 Input-Output Analysis 556
18.1.4 The Spatial Location of Population and Employment 557
18.2 Land-Use Transport Interaction Modelling 557
18.2.1 The Lowry Model 559
18.2.2 The Bid-Choice Model 560
18.2.2.1 Elasticities in Bid-Auction Location Choice Models 560
18.2.2.2 Consumer Surplus 561
18.2.3 Systems Dynamics Approach 561
18.2.4 Urban Simulation 563
18.3 Car-Ownership Forecasting 564
18.3.1 Background 564
18.3.2 Time-Series Extrapolations 565
18.3.3 Econometric Methods 568
18.3.3.1 The Method of Quarmby and Bates (1970) 568
18.3.3.2 The Regional Highway Transport Model (RHTM) Method 570
18.3.3.3 Models of Car Ownership and Use 570
18.3.3.4 Models of Motorcycle Ownership 571
18.3.4 International Comparisons 571
18.4 The Value of Travel Time 573
18.4.1 Introduction 573
18.4.2 Subjective and Social Values of Time 574
18.4.3 Some Practical Results 575
18.4.4 Methods of Analysis 576
18.4.4.1 Estimation of Subjective Values of Time 576
18.4.4.2 Confidence Intervals for the Value of Time 577
18.4.4.3 A Deeper Look at Computing Measures of Uncertainty for WTP 580
18.4.4.4 Special Problems Brought in by the Use of More Flexible Models 582
18.4.4.5 The Transfer Price Approach 589
18.4.4.6 The Stated Preference Approach 590
18.5 Valuing External Effects of Transport 590
18.5.1 Introduction 590
18.5.2 Methods of Analysis 591
18.5.2.2 Contingent Valuation 593
Exercises 596
19 Pricing and Revenue 599
19.1 Pricing and Welfare 599
19.2 Correcting Prices for Externalities 600
19.3 The Perception of Travel Costs 601
19.4 Pricing Tools 601
19.4.1 Car Ownership Taxes 602
19.4.2 Fuel Taxes 602
19.4.3 Parking Charges 602
19.4.4 Tolled Facilities 603
19.4.5 Pay-As-You-Drive Insurance 604
19.4.6 Congestion and Road User Charging 604
19.5 The Experience of Private Sector Projects 605
19.5.1 Involvement of Private Sector in Transport Projects 605
19.5.2 Uncertainty and Risk 607
19.5.3 Risk Management and Mitigation 609
19.6 Demand Modelling 610
19.6.1 Willingness to Pay 610
19.6.2 Simple Projects 610
19.6.3 Complex Projects 611
19.6.4 Road User Charge Projects 613
19.6.5 Scheme Design 613
19.6.6 Ramp-Up, Leakage, and Discounts 615
19.7 Risk Analysis 616
19.7.1 Sensitivity and Sources of Risk 617
19.7.2 Stochastic Risk Analysis 618
19.7.3 Scenarios 619
19.8 Conclusions 620
Exercises 620
20 Modelling the Less Common 623
20.1 Introduction 623
20.2 New Scheduled Services 624
20.3 Walking 625
20.4 Cycling 625
20.5 Motorcycling 627
20.6 Parking 628
20.7 Demand-Responsive Transport 630
20.7.1 Introduction 630
20.7.2 Micro-Mobility Sharing 633
20.7.3 Car, Motorcycle, and Van Sharing 634
20.7.4 Connected and Automated Vehicles 635
20.7.5 Mobility as a Service 636
20.8 Modelling Demand-Responsive Mobility 636
20.8.1 The Challenge 636
20.8.2 Modelling Approaches 638
20.8.3 Model Outputs 640
20.9 Deliveries and Collections 640
20.10 Digital and Distant Presence 641
20.11 Soft Measures, Smarter Choices 642
References 645
Index 689
Dr. Luis G. Willumsen is an internationally recognised authority in transport and traffic modelling and has over 30 years of experience in this area. He previously lectured at Leeds University and University College London, and was also a Director of Steer before leaving in 2009 to set up his own independent practice. He is also Managing Partner of Nommon Solutions and Technologies, a company processing big data to provide location and mobility intelligence.